Book Downloads Hub Reads Ebooks Online eBook Librarys Digital Books Store Download Book Pdfs Bookworm Downloads Book Library Help Epub Book Collection Pdf Book Vault Read and Download Books Open Source Book Library Best Book Downloads Ashley Adams Volker Hunecke Gary J Wolff Mohamed Lamine Yansane Bruno Walter Steve Ruis Chitra Soundar Franci Rabinek Epstein
Do you want to contribute by writing guest posts on this blog?
Please contact us and send us a resume of previous articles that you have written.
Improving Flood Prediction: Assimilating Uncertain Crowdsourced Data

Every year, floods wreak havoc on numerous communities around the world, causing massive destruction and loss of lives. To mitigate the impact of these disasters, accurate flood prediction models play a crucial role. However, the accuracy of these models heavily relies on the quality and quantity of the data that feeds into them.
Traditionally, flood prediction models have predominantly relied on data collected from various sensors and gauges. Although these sources provide invaluable information, they often have limited coverage, especially in remote or underdeveloped regions. This limitation contributes to uncertainties in flood predictions, leaving communities vulnerable and ill-prepared.
A potential solution to address this data gap lies within crowdsourcing. With the widespread usage and accessibility of smartphones, individuals can contribute to flood prediction by reporting and sharing real-time information about water levels, rainfall, and other relevant observations. This approach allows for a much broader coverage and can supplement existing data collected from traditional sources.
4.4 out of 5
Language | : | English |
File size | : | 31395 KB |
Screen Reader | : | Supported |
Print length | : | 240 pages |
The Value of Crowdsourced Data
Integrating crowdsourced data into flood prediction models presents numerous advantages. Firstly, it can enhance spatial coverage, especially in regions with limited or no sensor networks. By assimilating data from crowd reports, prediction models can generate more comprehensive flood risk assessments, enabling authorities to issue earlier warnings and evacuation orders.
Secondly, crowdsourcing data allows for near real-time updates. Traditional data sources often have latency issues, which can delay flood predictions. However, with user-generated reports, a continuous stream of fresh data becomes available, ensuring more accurate forecasts and timely response from emergency management teams.
Moreover, crowdsourced data can contribute to improving the accuracy of flood models in previously unmonitored areas. By incorporating this information, model algorithms can learn and adjust, refining their predictions over time.
Addressing Uncertainty
While crowdsourced data holds great potential in flood prediction, it also introduces challenges in terms of uncertainty. Unlike data collected from sensors and gauges, crowd reports can vary in their accuracy and reliability. Some contributors might submit erroneous or intentionally misleading information, or their observations may be affected by subjective biases.
To address these inherent uncertainties, researchers and scientists are developing methods to assess the quality and reliability of crowdsourced flood data. Approaches such as outlier detection algorithms and reputation systems can help identify potentially unreliable reports and filter out misleading information.
Additionally, machine learning techniques can be utilized to weigh the credibility of each report based on the contributor's historical reliability and accuracy. By incorporating these assessment mechanisms, flood prediction models can assimilate crowdsourced data in a more informed manner, reducing uncertainties and improving overall accuracy.
The Role of Technology
Advancements in technology are enabling the effective assimilation of crowdsourced data into flood prediction models. Mobile applications and platforms have been developed to facilitate the collection and sharing of user-generated flood reports.
Furthermore, geolocation features in smartphones allow for accurate positioning of flood observations, which can be crucial for identifying vulnerable areas and assessing the severity of potential flooding.
Integration with Geographic Information Systems (GIS) enables the visualization and analysis of crowdsourced data in conjunction with other relevant layers, such as elevation and land use. These applications enhance decision-making processes by providing a comprehensive understanding of the flood risk landscape.
The Future of Flood Prediction
As the adoption of crowdsourced data in flood prediction continues to grow, it is essential to foster collaborations between scientists, policymakers, and communities. This interdisciplinary approach can leverage the power of collective intelligence and ensure the successful assimilation of uncertain data.
Flood prediction models need to evolve to effectively accommodate the integration of crowdsourced data, machine learning algorithms, and other emerging technologies. By constantly refining and updating these models, we can enhance prediction accuracy, reducing the devastating impact of floods on vulnerable populations.
, the assimilation of uncertain crowdsourced data into flood prediction models has the potential to revolutionize our ability to forecast and prepare for flooding events. With proper validation, assessment, and utilization of this data, we can improve the accuracy and timeliness of flood predictions, ultimately saving lives and minimizing the destructive consequences of these natural disasters.
4.4 out of 5
Language | : | English |
File size | : | 31395 KB |
Screen Reader | : | Supported |
Print length | : | 240 pages |
In recent years, the continued technological advances have led to the spread of low-cost sensors and devices supporting crowdsourcing as a way to obtain observations of hydrological variables in a more distributed way than the classic static physical sensors. The main advantage of using these type of sensors is that they can be used not only by technicians but also by regular citizens. However, due to their relatively low reliability and varying accuracy in time and space, crowdsourced observations have not been widely integrated in hydrological and/or hydraulic models for flood forecasting applications. Instead, they have generally been used to validate model results against observations, in post-event analyses.
This research aims to investigate the benefits of assimilating the crowdsourced observations, coming from a distributed network of heterogeneous physical and social (static and dynamic) sensors, within hydrological and hydraulic models, in order to improve flood forecasting. The results of this study demonstrate that crowdsourced observations can significantly improve flood prediction if properly integrated in hydrological and hydraulic models. This study provides technological support to citizen observatories of water, in which citizens not only can play an active role in information capturing, evaluation and communication, leading to improved model forecasts and better flood management.
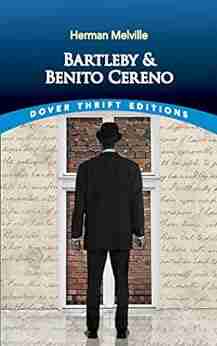

Unmasking the Enigma: A Colliding World of Bartleby and...
When it comes to classic literary works,...
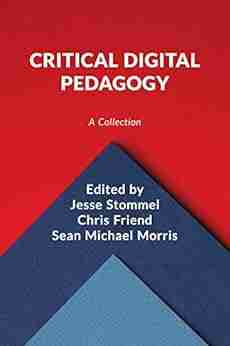

Critical Digital Pedagogy Collection: Revolutionizing...
In today's rapidly evolving digital...
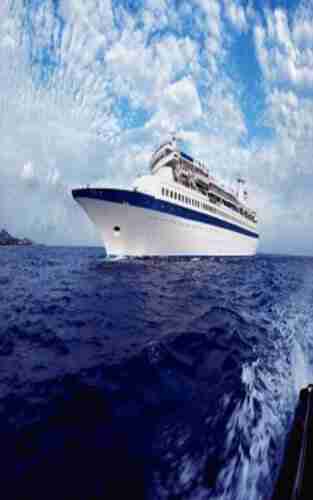

The Diary Of Cruise Ship Speaker: An Unforgettable...
Embark on an incredible...
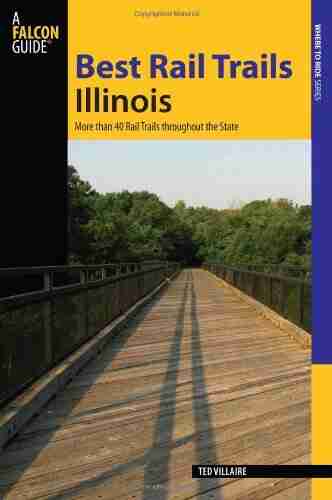

Best Rail Trails Illinois: Discover the Perfect Trails...
If you're an outdoor enthusiast looking...
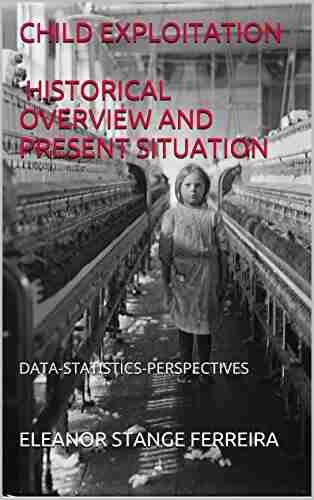

Child Exploitation: A Historical Overview And Present...
Child exploitation is a...
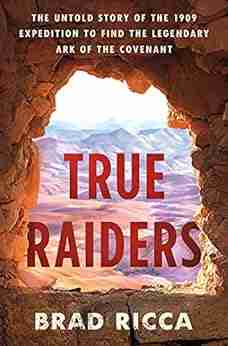

The Untold Story Of The 1909 Expedition To Find The...
Deep within the realms of legends and...
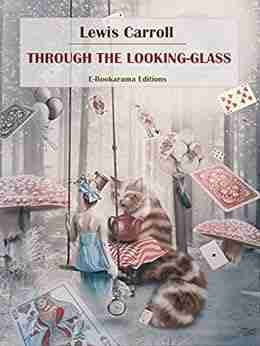

Through The Looking Glass - A Wonderland Adventure
Lewis Carroll,...
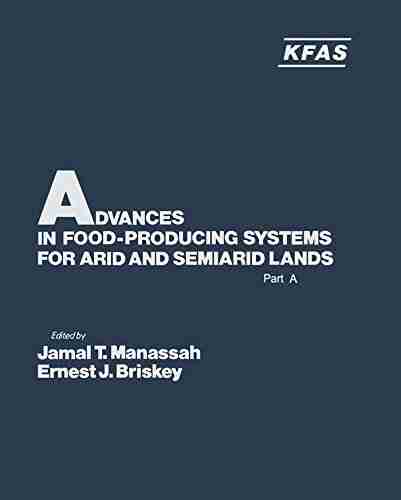

Advances In Food Producing Systems For Arid And Semiarid...
In the face of global warming and the...
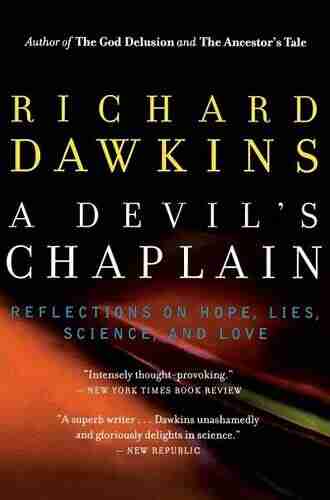

The Devil Chaplain: Exploring the Intriguing Duality of...
When it comes to the relationship between...
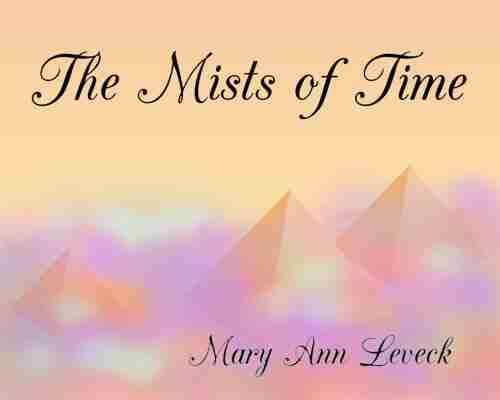

The Mists of Time: Cassie and Mekore - Unraveling the...
Have you ever wondered what lies beyond...
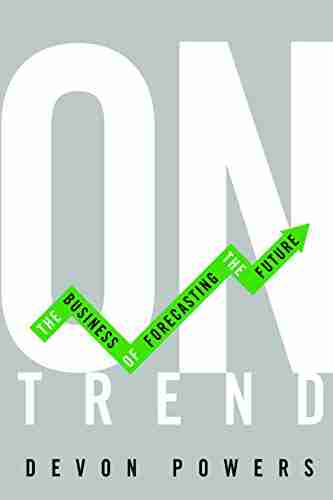

On Trend: The Business of Forecasting The Future
Do you ever wonder what the future holds?...
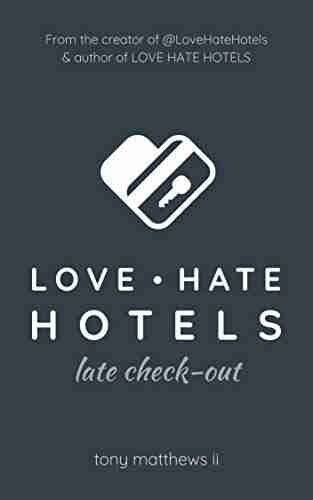

Love Hate Hotels Late Check Out
Have you ever experienced the joy of...
Light bulbAdvertise smarter! Our strategic ad space ensures maximum exposure. Reserve your spot today!
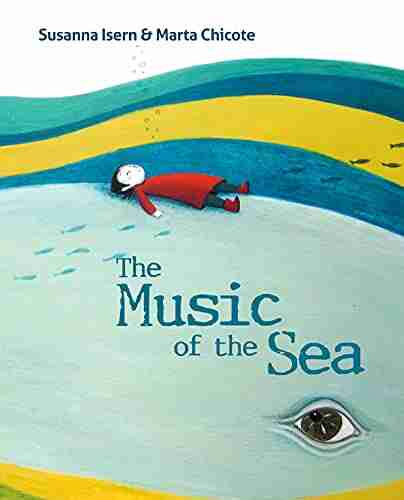

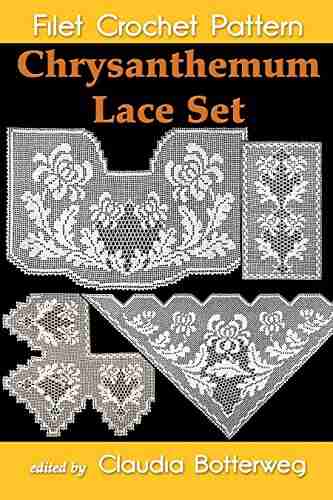

- Maurice ParkerFollow ·11.2k
- Cameron ReedFollow ·2.7k
- Jorge AmadoFollow ·14.9k
- Ken SimmonsFollow ·16.6k
- Ignacio HayesFollow ·11.4k
- Morris CarterFollow ·8.5k
- Jayson PowellFollow ·18.8k
- Fyodor DostoevskyFollow ·13.4k