Book Downloads Hub Reads Ebooks Online eBook Librarys Digital Books Store Download Book Pdfs Bookworm Downloads Book Library Help Epub Book Collection Pdf Book Vault Read and Download Books Open Source Book Library Best Book Downloads Thomas Sowell Richard Panchyk William Temple Hornaday Virgil William E Odom Daniel A Reed Patti Lawson Cindy Sellon
Do you want to contribute by writing guest posts on this blog?
Please contact us and send us a resume of previous articles that you have written.
The Secrets of Causality and Correlation Unveiled: How Artificial Intelligence Empowers Rational Decision Making

Are you tired of making decisions based on unfounded assumptions or incomplete information? Do you seek a powerful tool to enhance your decision-making process? Look no further! In this comprehensive guide, we delve into the fascinating concepts of causality, correlation, and how artificial intelligence (AI) revolutionizes rational decision making.
Understanding the Foundation: Causality vs. Correlation
Before we delve into the realm of AI, let's establish a clear distinction between causality and correlation. Causality refers to the relationship between cause and effect, where one event directly influences another. On the other hand, correlation represents a statistical relationship between two variables, suggesting a pattern without insinuating causation. It is vital to grasp this difference to make informed decisions and avoid falling victim to false assumptions.
The Rise of Artificial Intelligence in Decision Making
The exponential growth of AI presents a golden opportunity for rational decision making. By leveraging sophisticated algorithms and immense computing power, AI can analyze vast amounts of data, identifying intricate patterns and relationships that humans may overlook. Through this analysis, AI can uncover both causality and correlation, shedding light on the factors that underpin complex phenomena.
5 out of 5
Language | : | English |
File size | : | 3627 KB |
Text-to-Speech | : | Enabled |
Screen Reader | : | Supported |
Enhanced typesetting | : | Enabled |
Print length | : | 208 pages |
The Role of Machine Learning
Machine learning, a subfield of AI, plays a pivotal role in identifying causality and correlation. With access to extensive datasets, algorithms can learn and adapt, becoming more accurate over time. By recognizing patterns within the data, these algorithms can distinguish between causation and correlation, leading to more effective decision making.
Enhancing Decision Making Through Causality and Correlation
Incorporating causality and correlation into decision making enhances the precision and effectiveness of outcomes. By understanding the causal relationships behind events or phenomena, decision-makers can identify root causes, anticipate potential consequences, and make proactive choices. Furthermore, recognizing correlations enables decision-makers to consider related variables that can influence outcomes significantly.
The Role of AI in Causal Inference
AI excels in causal inference, the process of identifying the causal relationship between variables. Through machine learning algorithms, AI can analyze complex data and identify causal links that may not be immediately apparent. This empowers decision-makers to implement evidence-based strategies, leading to more favorable outcomes.
The Power of AI in Predictive Analytics
Predictive analytics, another area where AI thrives, leverages both causality and correlation to make accurate predictions. By analyzing historical data, AI algorithms can identify causal relationships and correlations, allowing decision-makers to foresee future trends and make data-driven decisions accordingly. Whether it's predicting market fluctuations or customer behavior, AI equips decision-makers with actionable insights.
Challenges and Ethical Considerations
While AI holds immense potential for enhancing rational decision making, it also poses several challenges. Ethical considerations are crucial, as biases in training data can lead to skewed results. Decision-makers must remain vigilant in ensuring fairness, transparency, and accountability while utilizing AI for decision making. Additionally, understanding the limitations and potential pitfalls of AI is essential to avoid overreliance and unintended consequences.
Embracing the Future of Decision Making
, the convergence of causality, correlation, and AI fuels the future of rational decision making. By harnessing the power of AI, decision-makers can leverage statistical relationships and uncover hidden causality, empowering them to make informed choices with increased precision. However, it is important to approach AI with caution and ethical considerations, ensuring fair, transparent, and accountable practices. Embrace the evolving landscape of decision making and unlock the potential of AI for a brighter future.
5 out of 5
Language | : | English |
File size | : | 3627 KB |
Text-to-Speech | : | Enabled |
Screen Reader | : | Supported |
Enhanced typesetting | : | Enabled |
Print length | : | 208 pages |
Causality has been a subject of study for a long time. Often causality is confused with correlation. Human intuition has evolved such that it has learned to identify causality through correlation. In this book, four main themes are considered and these are causality, correlation, artificial intelligence and decision making. A correlation machine is defined and built using multi-layer perceptron network, principal component analysis, Gaussian Mixture models, genetic algorithms, expectation maximization technique, simulated annealing and particle swarm optimization. Furthermore, a causal machine is defined and built using multi-layer perceptron, radial basis function, Bayesian statistics and Hybrid Monte Carlo methods. Both these machines are used to build a Granger non-linear causality model. In addition, the Neyman-Rubin, Pearl and Granger causal models are studied and are unified. The automatic relevance determination is also applied to extend Granger causality framework to the non-linear domain. The concept of rational decision making is studied, and the theory of flexibly-bounded rationality is used to extend the theory of bounded rationality within the principle of the indivisibility of rationality. The theory of the marginalization of irrationality for decision making is also introduced to deal with satisficing within irrational conditions. The methods proposed are applied in biomedical engineering, condition monitoring and for modelling interstate conflict.
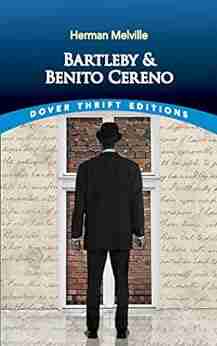

Unmasking the Enigma: A Colliding World of Bartleby and...
When it comes to classic literary works,...
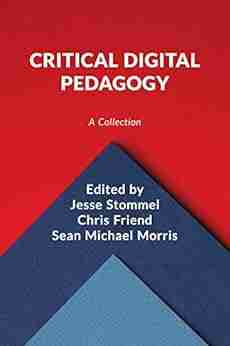

Critical Digital Pedagogy Collection: Revolutionizing...
In today's rapidly evolving digital...
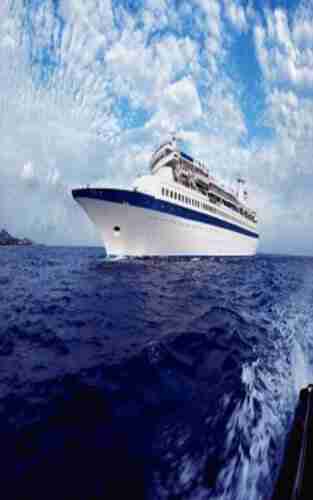

The Diary Of Cruise Ship Speaker: An Unforgettable...
Embark on an incredible...
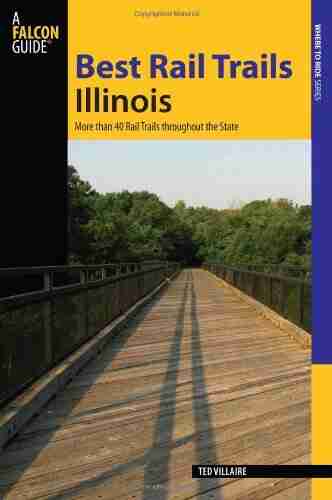

Best Rail Trails Illinois: Discover the Perfect Trails...
If you're an outdoor enthusiast looking...
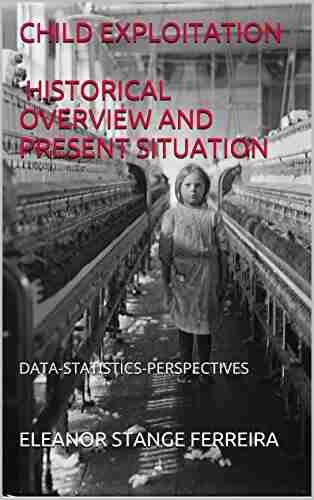

Child Exploitation: A Historical Overview And Present...
Child exploitation is a...
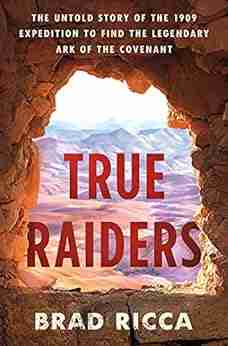

The Untold Story Of The 1909 Expedition To Find The...
Deep within the realms of legends and...
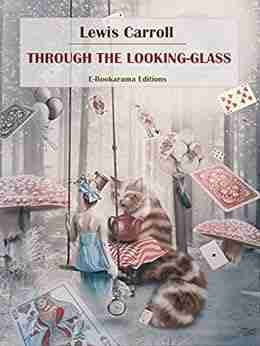

Through The Looking Glass - A Wonderland Adventure
Lewis Carroll,...
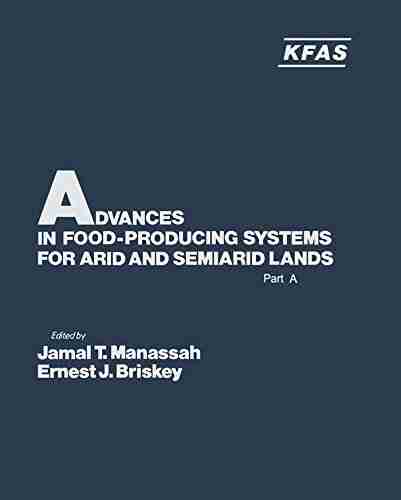

Advances In Food Producing Systems For Arid And Semiarid...
In the face of global warming and the...
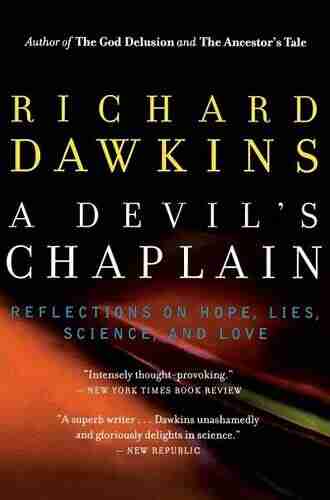

The Devil Chaplain: Exploring the Intriguing Duality of...
When it comes to the relationship between...
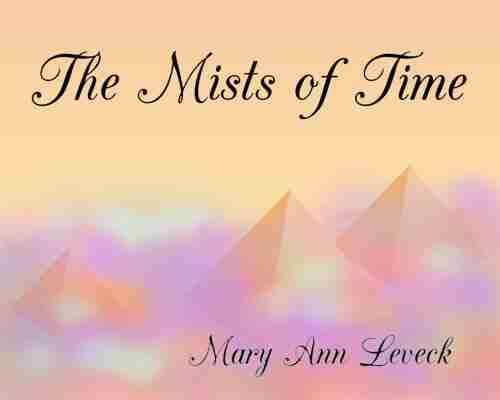

The Mists of Time: Cassie and Mekore - Unraveling the...
Have you ever wondered what lies beyond...
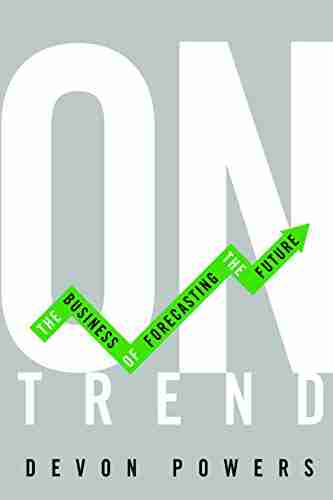

On Trend: The Business of Forecasting The Future
Do you ever wonder what the future holds?...
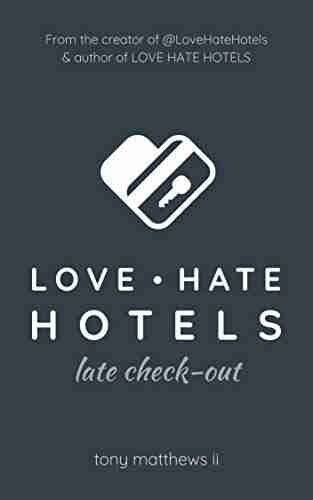

Love Hate Hotels Late Check Out
Have you ever experienced the joy of...
Light bulbAdvertise smarter! Our strategic ad space ensures maximum exposure. Reserve your spot today!
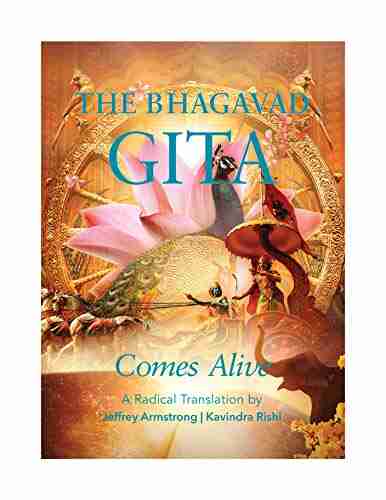

- Francisco CoxFollow ·9.5k
- Deion SimmonsFollow ·7.8k
- Jacques BellFollow ·12.5k
- Jackson BlairFollow ·15.2k
- Chase MorrisFollow ·2.1k
- Carl WalkerFollow ·17.7k
- Charlie ScottFollow ·12k
- Clay PowellFollow ·8.6k