Book Downloads Hub Reads Ebooks Online eBook Librarys Digital Books Store Download Book Pdfs Bookworm Downloads Book Library Help Epub Book Collection Pdf Book Vault Read and Download Books Open Source Book Library Best Book Downloads Roby Jose Ciju Joachim Salmann Rick Steves Jennifer Rudolph Walsh Robin Lane Fox Kingsley M Oliver The Editors Of Blue Shoe Press Jiapu Zhang
Do you want to contribute by writing guest posts on this blog?
Please contact us and send us a resume of previous articles that you have written.
Unlocking the Potential: Machine Learning for Evolution Strategies in Big Data

With the rise of big data, businesses and organizations are finding themselves faced with enormous amounts of information that can be difficult to analyze and extract meaningful insights from. Machine learning, a subfield of artificial intelligence, presents a solution to this challenge. In particular, machine learning algorithms can facilitate the use of evolution strategies to tackle big data problems.
Evolution Strategies and Big Data
Evolution strategies are a class of optimization algorithms inspired by biological evolution. These algorithms iteratively refine a population of candidate solutions, taking advantage of principles such as selection, recombination, and mutation to improve the solutions over time. They have been successfully applied to a wide range of optimization problems.
When it comes to big data, evolution strategies can play a crucial role in uncovering valuable patterns and trends. By utilizing machine learning techniques, these strategies can adapt and evolve over time to handle the vast amount of data available, leading to more accurate and efficient analyses.
4.5 out of 5
Language | : | English |
File size | : | 3743 KB |
Text-to-Speech | : | Enabled |
Enhanced typesetting | : | Enabled |
Print length | : | 179 pages |
Screen Reader | : | Supported |
Paperback | : | 27 pages |
Item Weight | : | 4.3 ounces |
Dimensions | : | 8.5 x 0.07 x 11 inches |
Benefits of Using Machine Learning in Evolution Strategies
Machine learning techniques have numerous advantages when combined with evolution strategies for big data studies. Some key benefits include:
1. Scalability
Machine learning algorithms can handle vast amounts of data in a scalable manner. This scalability ensures that evolution strategies can process and analyze big data sets efficiently.
2. Autonomous Learning
Machine learning algorithms can autonomously learn from the data they are exposed to. This allows evolution strategies to adapt and improve their performance over time without relying on manual adjustments.
3. Pattern Recognition
By using machine learning techniques, evolution strategies can identify complex patterns and correlations within big data sets. This enables businesses to make data-driven decisions and gain deeper insights into their operations.
4. Predictive Analytics
Machine learning algorithms are capable of building predictive models based on historical data. By integrating these models into evolution strategies, organizations can make accurate predictions about future trends and behaviors, leading to better planning and decision-making.
5. Real-Time Analysis
Machine learning algorithms can be trained to analyze big data in real-time. This allows evolution strategies to provide prompt insights and recommendations, enabling businesses to respond quickly to changing market conditions.
Challenges and Considerations
While the combination of machine learning and evolution strategies offers significant potential for big data studies, it is important to consider several challenges:
1. Data Quality
The accuracy and reliability of machine learning models heavily depend on the quality of the input data. It is crucial to ensure that the data used for analysis is clean, consistent, and representative of the problem at hand.
2. Algorithm Selection
There are various machine learning algorithms available, each suited for different tasks and data characteristics. Choosing the right algorithm is essential to obtain accurate and meaningful results.
3. Computational Resources
Performing machine learning and evolution strategies on big data requires considerable computational resources, including processing power and storage capacity. Organizations must invest in infrastructure to support these requirements.
4. Privacy and Security
Dealing with big data necessitates a careful approach to privacy and security. Maintaining data confidentiality and ensuring compliance with relevant regulations should be a top priority when conducting machine learning studies.
Machine learning, when combined with evolution strategies, holds tremendous potential for analyzing big data and extracting valuable insights. The scalability, adaptability, and predictive capabilities of machine learning algorithms make them a powerful tool in the field of big data analytics. However, it is crucial to address challenges such as data quality, algorithm selection, computational resources, and privacy considerations to ensure successful implementation. By leveraging these technologies and best practices, businesses and organizations can unlock new opportunities and gain a competitive edge in the era of big data.
4.5 out of 5
Language | : | English |
File size | : | 3743 KB |
Text-to-Speech | : | Enabled |
Enhanced typesetting | : | Enabled |
Print length | : | 179 pages |
Screen Reader | : | Supported |
Paperback | : | 27 pages |
Item Weight | : | 4.3 ounces |
Dimensions | : | 8.5 x 0.07 x 11 inches |
This book
introduces numerous algorithmic hybridizations between both worlds that show
how machine learning can improve and support evolution strategies. The set of
methods comprises covariance matrix estimation, meta-modeling of fitness and
constraint functions, dimensionality reduction for search and visualization of
high-dimensional optimization processes, and clustering-based niching. After
giving an to evolution strategies and machine learning, the book
builds the bridge between both worlds with an algorithmic and experimental
perspective. Experiments mostly employ a (1+1)-ES and are implemented in Python
using the machine learning library scikit-learn. The examples are conducted on
typical benchmark problems illustrating algorithmic concepts and their
experimental behavior. The book closes with a discussion of related lines of
research.
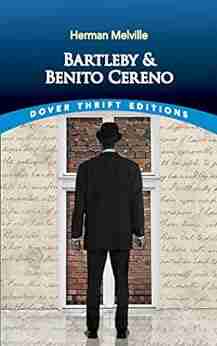

Unmasking the Enigma: A Colliding World of Bartleby and...
When it comes to classic literary works,...
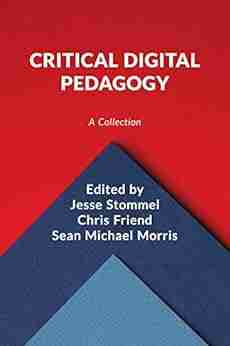

Critical Digital Pedagogy Collection: Revolutionizing...
In today's rapidly evolving digital...
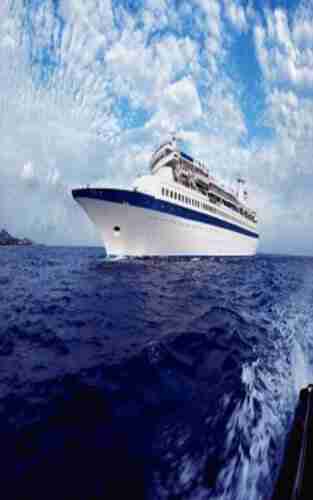

The Diary Of Cruise Ship Speaker: An Unforgettable...
Embark on an incredible...
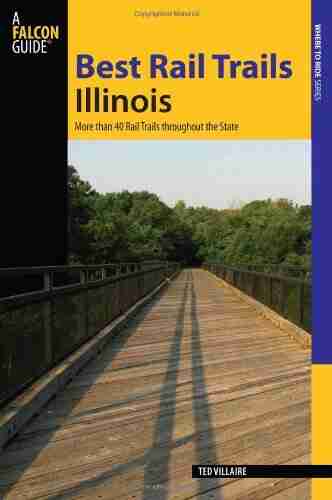

Best Rail Trails Illinois: Discover the Perfect Trails...
If you're an outdoor enthusiast looking...
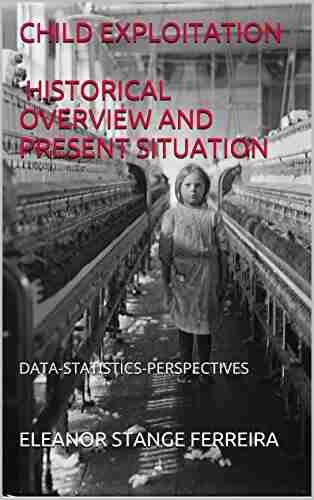

Child Exploitation: A Historical Overview And Present...
Child exploitation is a...
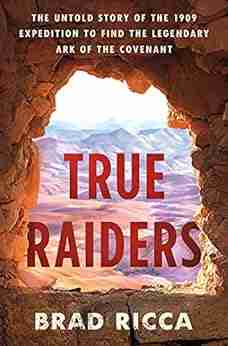

The Untold Story Of The 1909 Expedition To Find The...
Deep within the realms of legends and...
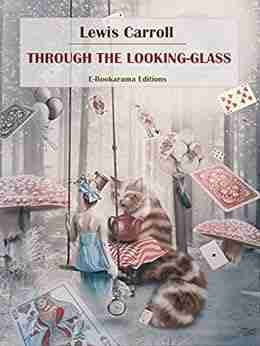

Through The Looking Glass - A Wonderland Adventure
Lewis Carroll,...
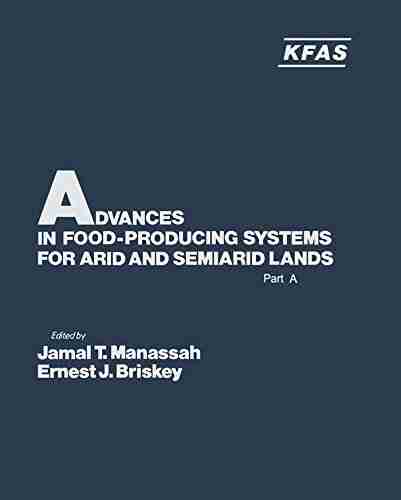

Advances In Food Producing Systems For Arid And Semiarid...
In the face of global warming and the...
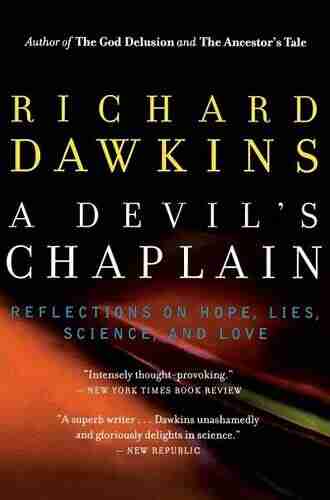

The Devil Chaplain: Exploring the Intriguing Duality of...
When it comes to the relationship between...
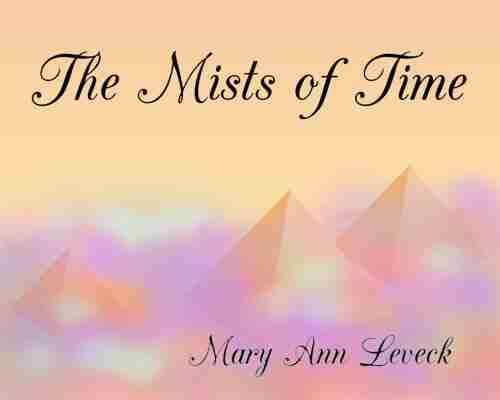

The Mists of Time: Cassie and Mekore - Unraveling the...
Have you ever wondered what lies beyond...
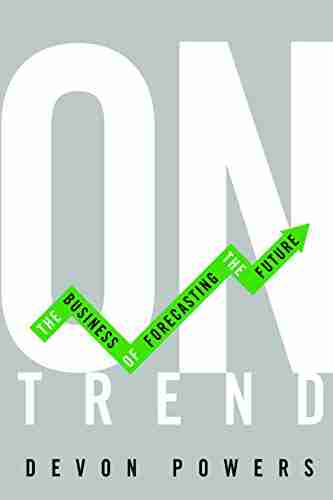

On Trend: The Business of Forecasting The Future
Do you ever wonder what the future holds?...
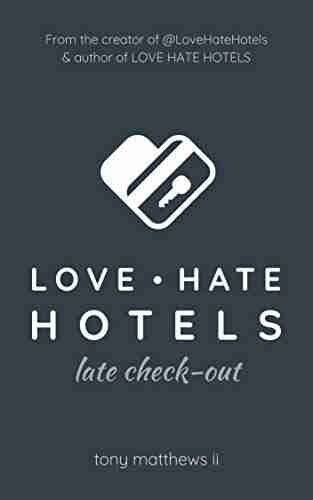

Love Hate Hotels Late Check Out
Have you ever experienced the joy of...
Light bulbAdvertise smarter! Our strategic ad space ensures maximum exposure. Reserve your spot today!
- Melvin BlairFollow ·9.1k
- Zadie SmithFollow ·11.7k
- Grant HayesFollow ·19.9k
- Gabriel HayesFollow ·18.2k
- Randy HayesFollow ·18.7k
- Wayne CarterFollow ·11.7k
- Leslie CarterFollow ·16.6k
- Edgar CoxFollow ·13.8k